Unlocking the Power of LangChain Parsers: Transform, Analyze, Simplify
Welcome to the Ultimate Guide on LangChain Parsers
Dive into the transformative world of LangChain Parsers, where complex data handling meets simplicity and efficiency. This page is your go-to resource for mastering LangChain's powerful parsing capabilities, including String Output Parsers, List Parsers, Regex Parsers, and Custom Output Parsers. Whether you're processing AI-generated text, structuring JSON data, or extracting insights with precision, LangChain Parsers provide the tools you need to streamline workflows and enhance your AI-driven applications. Explore in-depth examples, practical use cases, and easy-to-follow code snippets to unlock the full potential of these advanced parsers. Perfect for developers, data enthusiasts, and AI practitioners alike, this guide is tailored to help you transform, analyze, and simplify data effortlessly.
Let’s simplify AI parsing together!
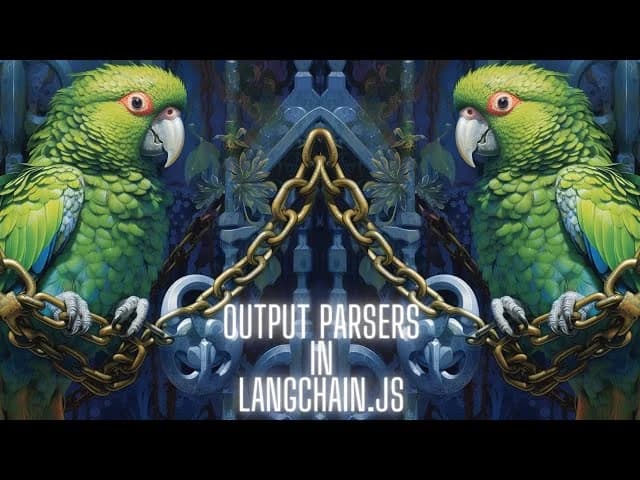
String Output Parser
This example demonstrates how to use the StringOutputParser from LangChain to process and parse AI-generated responses.
import { OpenAI } from "@langchain/openai";
import { StringOutputParser } from '@langchain/core/output_parsers'
import * as dotenv from "dotenv"
dotenv.config()
async function main() {
const model = new OpenAI({
modelName: "gpt-3.5-turbo",
temperature: 0.7,
openAIApiKey: process.env.OPENAI_API_KEY!
});
const parser = new StringOutputParser();
const prompt = "Provide a brief description of LangChain.";
const response = await model.call(prompt);
const parsedOutput = parser.parse(response);
console.log("Parsed Output:", parsedOutput);
}
main().catch(console.error);
By integrating OpenAI's GPT-3.5-turbo model, the script sends a prompt asking for a brief description of LangChain, retrieves the AI response, and uses the StringOutputParser to simplify and structure the output for easier handling and display. Perfect for developers looking to harness LangChain's parsing capabilities!
List Output Parser
This code defines a custom ListOutputParser class that parses a comma-separated string into an array.
import { OpenAI } from "@langchain/openai";
import { ListOutputParser } from "@langchain/core/output_parsers";
import * as dotenv from "dotenv";
dotenv.config();
// Custom implementation of ListOutputParser
class CustomListOutputParser extends ListOutputParser {
lc_namespace = ["custom", "parsers"];
async parse(text: string): Promise<string[]> {
try {
return text.split(",").map((item) => item.trim());
} catch {
throw new Error("Failed to parse response as a list.");
}
}
getFormatInstructions(): string {
return "Please respond with a comma-separated list of items. Example: Python, JavaScript, Java";
}
}
async function main() {
const model = new OpenAI({
modelName: "gpt-3.5-turbo",
temperature: 0.7,
openAIApiKey: process.env.OPENAI_API_KEY!,
});
const parser = new CustomListOutputParser();
const prompt = "List the top 5 programming languages used in AI development.";
const formattedPrompt = `${prompt}\n\n${parser.getFormatInstructions()}`;
try {
const response = await model.call(formattedPrompt);
const parsedOutput = await parser.parse(response);
console.log("Parsed Output:", parsedOutput);
} catch (error) {
const errorMessage = error instanceof Error ? error.message : "Unknown error occurred.";
console.error("Error:", errorMessage);
}
}
main().catch((error) =>
console.error("Unhandled Error:", error instanceof Error ? error.message : "Unknown error.")
);
It uses OpenAI's GPT-3.5-turbo model to generate responses guided by formatting instructions and processes the output into a structured list. The custom parser is necessary because ListOutputParser is an abstract class, requiring concrete implementation for methods like parse and properties like lc_namespace.
Why we cannot use ListOutputParser directly?
ListOutputParser is abstract and cannot be instantiated. It serves as a blueprint for creating custom parsers by enforcing implementation of required methods and properties. To use it on your webpage, a subclass with these implementations is necessary.
Structured Output Parser
This code demonstrates the use of a structured output parser to ensure the response from an OpenAI model is returned in a specific JSON format.
import { OpenAI } from "@langchain/openai";
import * as dotenv from "dotenv";
dotenv.config();
async function main() {
const model = new OpenAI({
modelName: "gpt-3.5-turbo",
temperature: 0.7,
openAIApiKey: process.env.OPENAI_API_KEY!,
});
const formatInstructions = `
Please provide the following information in JSON format:
{
"name": "string",
"description": "string",
"tags": ["string"]
}
`;
const prompt = `Provide information about LangChain in the specified format:\n${formatInstructions}\nLangChain is a framework for building AI applications.`;
const response = await model.call(prompt);
let parsedOutput;
try {
parsedOutput = JSON.parse(response);
if (
typeof parsedOutput.name === "string" &&
typeof parsedOutput.description === "string" &&
Array.isArray(parsedOutput.tags) &&
parsedOutput.tags.every((tag: unknown) => typeof tag === "string")
) {
console.log("Parsed Output:", parsedOutput);
} else {
throw new Error("Invalid format in the response.");
}
} catch (error: any) {
console.error("Error parsing or validating the response:", error.message);
}
}
main().catch(console.error);
The parser validates the model's response, enforcing data consistency by checking the types of provided fields such as `name`, `description`, and `tags`. This approach ensures clean and predictable outputs for downstream processing, showcasing the versatility of LangChain's integration for structured data workflows. Ideal for developers seeking robust data parsing in AI-driven applications, this example combines simplicity with precision in handling API responses.
Regex in LangChain
Learn how to extract structured data using regex in LangChain!
import { OpenAI } from "@langchain/openai";
import * as dotenv from "dotenv";
dotenv.config();
async function main() {
const model = new OpenAI({
modelName: "gpt-3.5-turbo",
temperature: 0.7,
openAIApiKey: process.env.OPENAI_API_KEY!,
});
// Define a regex pattern to extract name and age
const regexPattern = /Name:s*([ws]+)s*Age:s*(d+)/;
const prompt = "Provide a fictional character's name and age in the format: 'Name: [name] Age: [age]'.";
const response = await model.call(prompt);
// Apply regex matching manually
const match = response.match(regexPattern);
if (match) {
const parsedOutput = {
name: match[1]?.trim(),
age: match[2]?.trim(),
};
console.log("Parsed Output:", parsedOutput);
} else {
console.error("Failed to parse output with the given regex.");
}
}
main().catch(console.error);
This example demonstrates a manual approach to parsing AI-generated outputs, such as fictional characters' names and ages, into structured objects. Avoiding dependency-specific limitations, this method ensures compatibility and ease of use for a wide range of JavaScript and TypeScript applications. Perfect for parsing formatted AI responses with precision!
Comma-Separated List Output Parser
Discover how to leverage LangChain's CommaSeparatedListOutputParser to structure AI responses into lists for efficient handling.
import { OpenAI } from "@langchain/openai";
import { CommaSeparatedListOutputParser } from "@langchain/core/output_parsers";
import * as dotenv from "dotenv";
dotenv.config();
async function main() {
const model = new OpenAI({
modelName: "gpt-3.5-turbo",
temperature: 0.7,
openAIApiKey: process.env.OPENAI_API_KEY!,
});
const parser = new CommaSeparatedListOutputParser();
const prompt = "List three popular JavaScript frameworks, separated by commas.";
const response = await model.call(prompt);
const parsedOutput = parser.parse(response);
console.log("Parsed Output:", parsedOutput);
}
main().catch(console.error);
This example demonstrates how to integrate OpenAI's GPT-3.5-turbo model to generate a comma-separated list of JavaScript frameworks, and then seamlessly parse the response for immediate use in your applications. Perfect for developers seeking to streamline data extraction in natural language processing tasks.
Custom Output Parser
Unlock the Potential of Custom Output Parsers in LangChain Dive into the world of LangChain with this hands-on example of a Custom JSON Output Parser.
import { OpenAI } from "@langchain/openai";
import { BaseOutputParser } from "@langchain/core/output_parsers";
import * as dotenv from "dotenv";
dotenv.config();
class JSONOutputParser extends BaseOutputParser<any> {
lc_namespace = ["custom", "parsers"];
parse(input: string): any {
try {
return JSON.parse(input);
} catch (error: any) {
throw new Error(`Failed to parse JSON: ${error.message}`);
}
}
getFormatInstructions(): string {
return "Please respond with a valid JSON object. Ensure the syntax is correct.";
}
}
async function main() {
const model = new OpenAI({
modelName: "gpt-3.5-turbo",
temperature: 0.7,
openAIApiKey: process.env.OPENAI_API_KEY!,
});
const parser = new JSONOutputParser();
const prompt = `Describe a programming language in JSON format with fields for "name", "paradigm", and "popularFrameworks".`;
const formattedPrompt = `${prompt}\n\n${parser.getFormatInstructions()}`;
try {
const response = await model.call(formattedPrompt);
console.log("Raw Response:", response);
const parsedOutput = parser.parse(response.trim());
console.log("Parsed Output:", parsedOutput);
} catch (error) {
console.error("Error:", error.message);
}
}
main().catch(console.error);
Learn how to structure your AI responses into usable, structured JSON formats for seamless integration into your applications. This example showcases a practical implementation using LangChain’s powerful output parser base class, helping you transform raw text outputs into precise, structured data. Whether you're building APIs, chatbots, or data-driven apps, this example will elevate your AI parsing capabilities.
Conclusion
LangChain's parsers offer unparalleled flexibility for developers aiming to handle AI-driven data effectively. From simple text transformations to advanced structured JSON responses, these tools streamline data handling processes, ensuring clarity and precision. By incorporating LangChain's parsers into your workflow, you can enhance your AI-powered applications, improving both efficiency and scalability. This page serves as a comprehensive resource for developers to understand and utilize these powerful tools, paving the way for innovative data-driven solutions.