LangChain in Action: How to Build Intelligent AI Applications Easily and Efficiently ?
Mastering LangChain Retrievals: A Guide to Intelligent Information Access
In the realm of AI-powered applications, retrievals enable seamless access to relevant information from vast collections of unstructured data, such as documents, PDFs, or databases. Instead of treating every query as an isolated event, retrieval mechanisms in LangChain intelligently extract and utilize contextually relevant data to generate more accurate and insightful responses. By leveraging Retrieval Chains, LangChain integrates language models with retrieval systems, creating a powerful synergy for handling complex document interactions.
Use Cases for Retrievals
- Document Search and Summarization: Efficiently find and summarize key information from large document repositories, such as research papers or legal documents.
- Knowledge-Driven Chatbots: Build chatbots capable of answering specific user queries based on company documentation, product manuals, or FAQs.
- Healthcare Record Analysis: Extract insights from patient records to assist medical professionals in diagnosing conditions or reviewing histories.
- E-Learning Platforms: Create personalized study tools by retrieving content from educational materials based on user input.
- Customer Support Automation: Automate support workflows by retrieving relevant troubleshooting steps or policies from internal knowledge bases.
LangChain Retrieval Chains streamline these use cases by allowing applications to retrieve the most relevant information and use it as input for generating meaningful and context-aware responses. This approach not only enhances the efficiency and accuracy of retrieval but also enables the creation of more intelligent and adaptive AI systems.
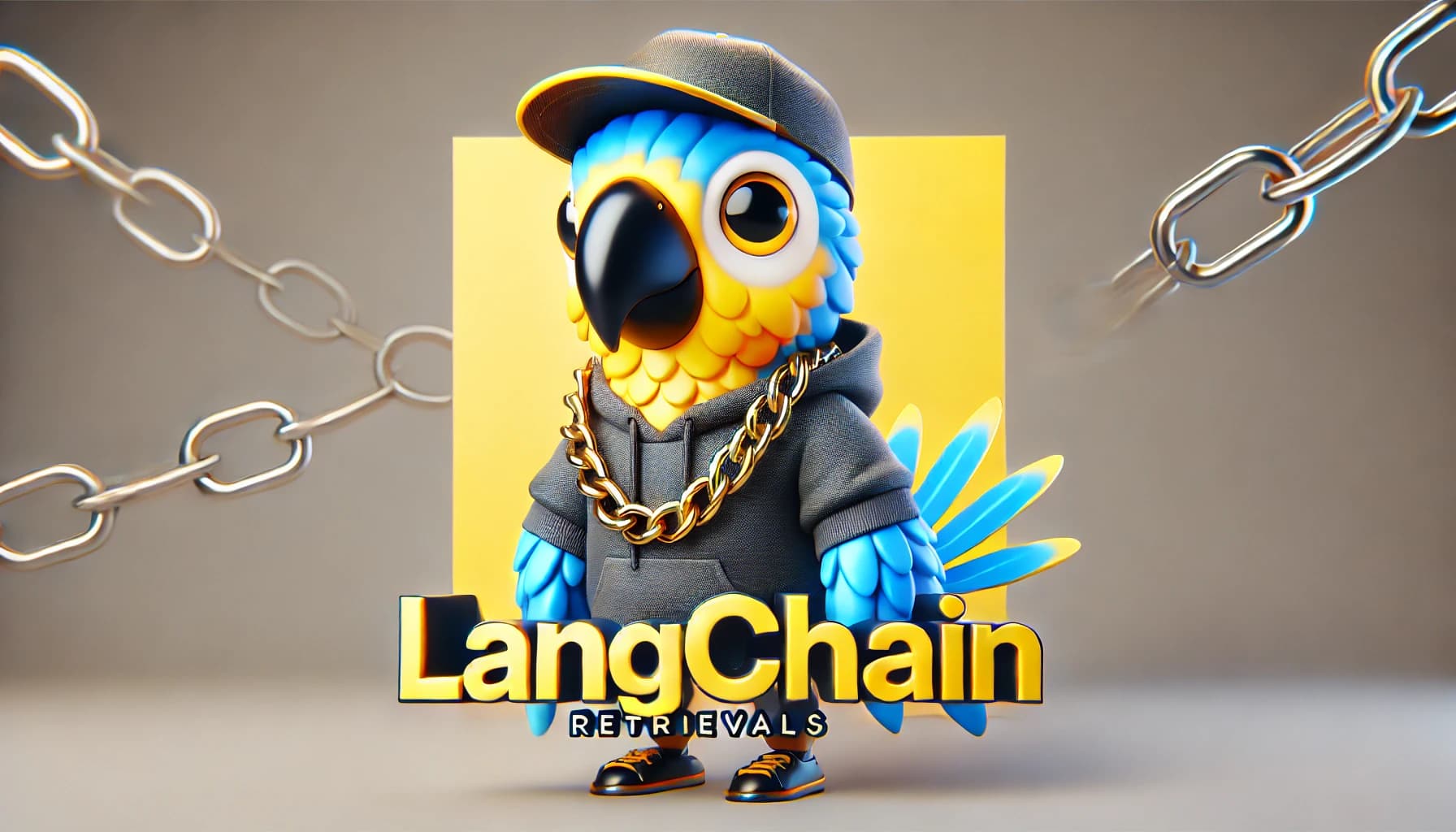
Document Querying: Using a Vector Store for Retrieval-Augmented Generation (RAG) with LangChain
This code demonstrates how to use LangChain with OpenAI’s ChatOpenAI model and OpenAIEmbeddings to implement Retrieval-Augmented Generation (RAG). Instead of relying solely on the AI model's pretrained knowledge, this approach enables dynamic retrieval of relevant information from a custom text dataset stored in a vector store. A vector store allows for efficient similarity search, meaning when a user asks a question, the system retrieves the most relevant text passages and uses them to generate a more accurate response. This method is particularly useful in chatbots, document search applications, and AI assistants that need context-aware responses.
╔════════════════════════════════════════════════════════════════════════════╗
║ 🌟 EDUCATIONAL EXAMPLE 🌟 ║
║ ║
║ 📌 This is a minimal and short working example for educational purposes. ║
║ ⚠️ Not optimized for production! ║
║ ║
║ 📦 Versions Used: ║
║ - "@langchain/core": "^0.3.38" ║
║ - "@langchain/openai": "^0.4.2" ║
║ ║
║ 🔄 Note: LangChain is transitioning from a monolithic structure to a ║
║ modular package structure. Ensure compatibility with future updates. ║
╚════════════════════════════════════════════════════════════════════════════╝
import { ChatOpenAI } from "@langchain/openai"; // import the ChatOpenAI class
import { OpenAIEmbeddings } from "@langchain/openai"; // import the OpenAIEmbeddings class
import { MemoryVectorStore } from "langchain/vectorstores/memory"; // import the MemoryVectorStore class
import dotenv from "dotenv"; // import the dotenv package
import fs from "fs/promises"; // import the fs/promises package
import { Document } from "@langchain/core/documents";
dotenv.config(); // load environment variables from .env file
// Initialize the ChatOpenAI model with the required parameters
const model = new ChatOpenAI({
modelName: "gpt-3.5-turbo",
temperature: 0.7,
openAIApiKey: process.env.OPENAI_API_KEY!,
});
//const embeddings = new OpenAIEmbeddings({ openAIApiKey: process.env.OPENAI_API_KEY! }); // Initialize the OpenAIEmbeddings class with the required parameters
const embeddings = new OpenAIEmbeddings({
modelName: "text-embedding-3-small", // or "text-embedding-3-large"
openAIApiKey: process.env.OPENAI_API_KEY!,
});
console.log("Embeddings:", embeddings.model); // log the embeddings
async function run() {
const filePath = "src/sample.txt"; // specify the path to the file containing the text to be processed
const fileContent = await fs.readFile(filePath, "utf8"); // read the file content
const splitDocuments = fileContent.split("
").map(line => line.trim()).filter(Boolean); // split the file content into an array of strings, filtering out any empty strings
const docs = splitDocuments.map((text, idx) => new Document({ pageContent: text, metadata: { id: idx }, id: String(idx) }));
const vectorStore = await MemoryVectorStore.fromDocuments(docs, embeddings);
// Initialize the MemoryVectorStore class with the required parameters
// const vectorStore = await MemoryVectorStore.fromTexts(
// splitDocuments,
// splitDocuments.map((_, idx) => ({ id: idx })),
// embeddings
// );
const retriever = vectorStore.asRetriever({ searchType: "similarity", k: 2 }); // Initialize the retriever with the required parameters
const userQuery = "What is LangChain?"; // specify the user query
const retrievedDocs = await retriever.invoke(userQuery); // retrieve the documents that match the user query
const context = retrievedDocs.map((doc) => doc.pageContent).join(""); // join the retrieved documents into a single string
const prompt = `Based on the following information, answer the question:${context} Question: ${userQuery}`; // construct the prompt
//console.log("🧾 Prompt to model:", prompt);
const response = await model.invoke(prompt); // invoke the model with the prompt
console.log("Retrieved Documents:", retrievedDocs);
console.log("Response:", response.content); // log the response
}
run().catch(console.error); // call the run function and handle any errors
This implementation follows these key steps:
- Initialize the AI Model & Embeddings: The ChatOpenAI model is loaded, and OpenAIEmbeddings is used to create numerical representations (vectors) of the text data.
- Load & Process Text Data: A text file (sample.txt) is read and split into smaller document chunks.
- Retrieve Relevant Documents: When the user asks a question ("What is LangChain?"), the system searches for the most similar text snippets using semantic similarity.
- Generate a Response: The retrieved text is used as context in a prompt, ensuring that the AI provides an informed and relevant answer rather than relying purely on its training data.
This approach makes AI applications more dynamic, context-aware, and able to reference up-to-date information, making it ideal for knowledge bases, FAQ bots, customer support systems, and research tools.
MemoryVectorStore in LangChain: All You Need to Know
MemoryVectorStore is a simple, in-memory vector database that supports document storage, embedding, and search. It’s best used for prototyping, testing, and small-scale applications where persistence isn’t needed.
Method | Description |
---|---|
addDocuments(documents) | Adds Document instances to the store. |
addVectors(vectors, documents) | Adds raw vectors alongside documents. Useful for adding pre-computed embeddings. |
similaritySearch(query, k) | Performs a similarity search and returns top-k matching documents. |
similaritySearchWithScore(query, k) | Like similaritySearch but includes similarity scores. |
maxMarginalRelevanceSearch(query, k, fetchK) | Diversified search using MMR (Good for diverse results). |
asRetriever(options) | This function transforms the vector store into a retriever that LangChain can use to extract relevant information based on a query. |
delete() | (Depends on store) Used for removing documents or vectors. |
Using .asRetriever()
The .asRetriever() method turns your vector store into a retriever interface that LangChain chains can consume. It supports flexible options like:
- 🔥 searchType: Determines how the retriever will perform the search.
- 🔥 "similarity": Returns the top-k most similar documents using vector distance.
- 🔥 "mmr": Applies Max Marginal Relevance to promote diversity in the results.
- 🔥 k: Number of documents to return.
- 🔥 fetchK (only for mmr): Number of documents to initially fetch before selecting the best k.
Similarity vs. MMR — What’s the Difference?
- 🔥 Similarity Search: Returns documents purely based on how close they are to the query in vector space (e.g., cosine similarity). Great for precision.
- 🔥 MMR (Max Marginal Relevance): Balances relevance with novelty. Ideal when you want diverse results and want to avoid duplicates or overly similar answers.
Example: If your query is "JavaScript frameworks", similarity search might return multiple results about React, while MMR could return results about React, Vue, Angular, and Svelte.
When Should You Use MemoryVectorStore?
Use it when you:
- 🔥 Need a fast, in-memory store for testing or small datasets.
- 🔥 Want to prototype a retrieval-based app without setting up external vector DBs.
- 🔥 Are working on educational or proof-of-concept projects.
For production workloads, you’ll probably want to upgrade to a persistent store like Qdrant, Pinecone, or Weaviate.
MemoryVectorStore is an excellent entry point into vector-based retrieval workflows in LangChain. It’s simple, fast, and plays well with other LangChain components — especially when paired with .asRetriever() and integrated into chains or agents.
If you're new to retrieval-augmented generation (RAG), this is a great place to start.
API Retrievals: Using OpenAI to Extract Information from API Data
This script demonstrates how to fetch data from an external API, process it using OpenAI’s gpt-3.5-turbo model, and generate structured responses. This approach is useful for data analysis, automated summarization, and chatbot applications where structured information needs to be extracted and reformatted. Whether you're building an AI-powered assistant, a customer support bot, or a research tool, this method allows for intelligent data extraction and streamlined information delivery.
╔════════════════════════════════════════════════════════════════════════════╗
║ 🌟 EDUCATIONAL EXAMPLE 🌟 ║
║ ║
║ 📌 This is a minimal and short working example for educational purposes. ║
║ ⚠️ Not optimized for production! ║
║ ║
║ 📦 Versions Used: ║
║ - "@langchain/core": "^0.3.38" ║
║ - "@langchain/openai": "^0.4.2" ║
║ ║
║ 🔄 Note: LangChain is transitioning from a monolithic structure to a ║
║ modular package structure. Ensure compatibility with future updates. ║
╚════════════════════════════════════════════════════════════════════════════╝
import { ChatOpenAI } from "@langchain/openai"; // Import the ChatOpenAI class used for chat-based models
import { ChatPromptTemplate } from "@langchain/core/prompts"; // Import the ChatPromptTemplate class used for chat-based prompts
import dotenv from "dotenv"; // Import the dotenv package
import readline from "readline"; // Import the readline package used for user input
dotenv.config(); // Load environment variables from the .env file
const rl = readline.createInterface({
input: process.stdin,
output: process.stdout,
}); // Create a readline interface for user input
const model = new ChatOpenAI({
modelName: "gpt-3.5-turbo",
temperature: 0.7,
openAIApiKey: process.env.OPENAI_API_KEY,
}); // Create a new ChatOpenAI instance with the required parameters
// Define a function to fetch data from an API using fetch
const fetchFromAPI = (endpoint: string) =>
fetch(endpoint)
.then((res) =>
res.ok ? res.json() : Promise.reject(`HTTP error! Status: ${res.status}`)
)
.catch((err) => console.error("Error fetching data:", err)); // Define a function to fetch data from an API
async function run() {
// Prompt the user for input
rl.question(
"Enter a user ID to fetch posts for user ID: ",
async (userId) => {
const apiData = await fetchFromAPI(
`https://jsonplaceholder.typicode.com/posts?userId=${userId}`
); // Fetch data from the API
if (!apiData?.length) return console.log("No data found."), rl.close(); // Check if the API data is empty
const prompt = await ChatPromptTemplate.fromMessages([
[
"system",
"You are a creative assistant that extracts information from API data.",
], // Create system message
["human", "Show these posts: {apiData}"], // Create human message
]).formatMessages({ apiData: JSON.stringify(apiData, null, 2) }); // Create a ChatPromptTemplate with the API data
console.log(
`[ASSISTANT]: ${
(await model.generate([prompt])).generations[0][0].text
}`
); // Generate a summary using the ChatOpenAI model
rl.close(); // Close the readline interface
}
);
}
run().catch(console.error); // Call the run function and handle any errors
Code Explanation
- Fetches posts from an API using a given userId.
- Formats the API data into a structured prompt using ChatPromptTemplate.
- Uses OpenAI’s model to process and summarize the retrieved data.
- Outputs the AI-generated response in a user-friendly format.
The script is useful for building chatbots, AI assistants, or automated reporting tools that require real-time data processing and response generation.
Using MongoDB and OpenAI for Job Search with Vector Similarity
In the modern job market, retrieving relevant job listings efficiently is crucial. This script integrates MongoDB with OpenAI embeddings to create an intelligent job search system using vector similarity search. The script allows users to enter a job title or keyword, and retrieves the most relevant job listings based on their input.
The goal is to:
- Fetch job listings from a MongoDB database.
- Convert job descriptions into vector embeddings using OpenAI.
- Perform similarity-based searches to find the most relevant jobs based on user input.
This approach is ideal for job search engines, recommendation systems, and AI-driven filtering tools where semantic search is more effective than basic keyword matching.
╔════════════════════════════════════════════════════════════════════════════╗
║ 🌟 EDUCATIONAL EXAMPLE 🌟 ║
║ ║
║ 📌 This is a minimal and short working example for educational purposes. ║
║ ⚠️ Not optimized for production! ║
║ ║
║ 📦 Versions Used: ║
║ - "@langchain/core": "^0.3.38" ║
║ - "@langchain/openai": "^0.4.2" ║
║ ║
║ 🔄 Note: LangChain is transitioning from a monolithic structure to a ║
║ modular package structure. Ensure compatibility with future updates. ║
╚════════════════════════════════════════════════════════════════════════════╝
import { MongoClient } from "mongodb"; // import MongoDB driver
import { OpenAIEmbeddings } from "@langchain/openai"; // import OpenAI embeddings
import { MemoryVectorStore } from "langchain/vectorstores/memory"; // import MemoryVectorStore for in-memory vector store
import dotenv from "dotenv"; // import dotenv
import readline from "readline"; // import readline for console input
dotenv.config(); // load environment variables
const MONGO_URI = process.env.MONGO_URI || "mongodb://localhost:27017"; // MongoDB connection URI
const DATABASE_NAME = "jobsbg"; // MongoDB database name
const COLLECTION_NAME = "jobs"; // MongoDB collection name
const rl = readline.createInterface({ input: process.stdin, output: process.stdout }); // create readline interface for console input
// function to connect to MongoDB
const connectToMongoDB = async () => {
const client = new MongoClient(MONGO_URI);
await client.connect();
console.log("✅ Connected to MongoDB");
return { client, collection: client.db(DATABASE_NAME).collection(COLLECTION_NAME) };
};
// function to fetch documents from MongoDB
const fetchMongoDBDocuments = async () => {
const { client, collection } = await connectToMongoDB(); // connect to MongoDB
const documents = await collection.find({}).toArray(); // fetch all documents
await client.close(); // close MongoDB connection
console.log("🔌 MongoDB connection closed.");
return documents; // return fetched documents
};
// function to retrieve documents
const retrieveDocuments = async (query: string) => {
const documents = await fetchMongoDBDocuments(); // fetch documents
if (!documents.length) return console.log("❌ No documents found in MongoDB."), []; // if no documents found
const vectorStore = await MemoryVectorStore.fromTexts(
documents.map(doc => `${doc.title} ${doc.description}`), // map documents to texts
documents.map(doc => ({ id: doc._id.toString(), title: doc.title })), // map documents to metadata
new OpenAIEmbeddings() // create OpenAI embeddings
);
console.log(`🔍 Searching for: "${query}"`);
return await vectorStore.similaritySearch(query, 2); // search for documents
};
async function run() {
// prompt user for input
rl.question("🔹 Enter a job title or keyword to search: ", async (query) => {
console.log("📄 Retrieved Documents:", JSON.stringify(await retrieveDocuments(query), null, 2)); // print retrieved documents
rl.close(); // close readline interface
});
};
run().catch(console.error); // catch errors
How the Code Works
This script connects to MongoDB, retrieves job listings, embeds the job descriptions as vectors, and searches for the most relevant jobs based on user input.
- Database Connection & Data Retrieval The script first connects to MongoDB using the MongoDB Node.js driver and fetches all job listings from the jobs collection. The job documents contain titles and descriptions.
- Embedding & Vector Storage The retrieved job listings are converted into text embeddings using OpenAIEmbeddings. These embeddings are stored in a MemoryVectorStore, an in-memory vector database that enables fast similarity search.
- Searching for Similar Jobs The user inputs a job title or keyword. The system retrieves the top 2 most relevant jobs using vector similarity search. Instead of simple keyword matching, the search considers semantic meaning.
- Displaying the Results The retrieved job listings are displayed in the console. The MongoDB connection is closed properly after fetching the data.
This approach ensures smarter, context-aware job retrieval instead of basic keyword searches.
Multi Query Retriever
Enhances search coverage by generating multiple reformulations of the original question, increasing the chances of retrieving relevant results.
╔════════════════════════════════════════════════════════════════════════════╗
║ 🌟 EDUCATIONAL EXAMPLE 🌟 ║
║ ║
║ 📌 This is a minimal and short working example for educational purposes. ║
║ ⚠️ Not optimized for production! ║
║ ║
║ 📦 Versions Used: ║
║ - "@langchain/core": "^0.3.38" ║
║ - "@langchain/openai": "^0.4.2" ║
║ ║
║ 🔄 Note: LangChain is transitioning from a monolithic structure to a ║
║ modular package structure. Ensure compatibility with future updates. ║
╚════════════════════════════════════════════════════════════════════════════╝
import { ChatOpenAI } from "@langchain/openai";
import { MultiQueryRetriever } from "langchain/retrievers/multi_query";
import { MemoryVectorStore } from "langchain/vectorstores/memory";
import { OpenAIEmbeddings } from "@langchain/openai";
import { Document } from "@langchain/core/documents";
import * as dotenv from "dotenv";
dotenv.config();
// Sample documents
const documents = [
new Document({ pageContent: "Einstein developed the theory of relativity." }),
new Document({ pageContent: "Marie Curie was the first woman to win a Nobel Prize." }),
new Document({ pageContent: "Quantum physics explores atomic and subatomic systems." }),
new Document({ pageContent: "Relativity explains how time and space are linked." }),
];
async function run() {
// Step 1: Create vector store
const vectorStore = await MemoryVectorStore.fromDocuments(documents, new OpenAIEmbeddings());
// Step 2: Define base LLM
const llm = new ChatOpenAI({
modelName: "gpt-3.5-turbo",
temperature: 0,
openAIApiKey: process.env.OPENAI_API_KEY!,
});
// Step 3: Create MultiQueryRetriever
const retriever = MultiQueryRetriever.fromLLM({
llm,
retriever: vectorStore.asRetriever(),
});
// Step 4: Ask a natural language question
const question = "Who contributed to the theory of time and space?";
// Step 5: Retrieve relevant documents
const results = await retriever.invoke(question);
console.log("
📄 Relevant Results:");
results.forEach((doc, i) => {
console.log(`
#${i + 1}: ${doc.pageContent}`);
});
}
run().catch(console.error);
Contextual Compression Retriever
Applies compression or filtering techniques to the retrieved documents to return only the most essential and relevant information to the model.
╔════════════════════════════════════════════════════════════════════════════╗
║ 🌟 EDUCATIONAL EXAMPLE 🌟 ║
║ ║
║ 📌 This is a minimal and short working example for educational purposes. ║
║ ⚠️ Not optimized for production! ║
║ ║
║ 📦 Versions Used: ║
║ - "@langchain/core": "^0.3.38" ║
║ - "@langchain/openai": "^0.4.2" ║
║ ║
║ 🔄 Note: LangChain is transitioning from a monolithic structure to a ║
║ modular package structure. Ensure compatibility with future updates. ║
╚════════════════════════════════════════════════════════════════════════════╝
import { ChatOpenAI } from "@langchain/openai";
import { OpenAIEmbeddings } from "@langchain/openai";
import { MemoryVectorStore } from "langchain/vectorstores/memory";
import { ContextualCompressionRetriever } from "langchain/retrievers/contextual_compression";
import { LLMChainExtractor } from "langchain/retrievers/document_compressors/chain_extract";
import { Document } from "@langchain/core/documents";
import * as dotenv from "dotenv";
dotenv.config();
async function run() {
// Step 1: Sample documents
const docs = [
new Document({ pageContent: "Isaac Newton formulated the laws of motion and universal gravitation." }),
new Document({ pageContent: "Nikola Tesla worked on alternating current electrical systems." }),
new Document({ pageContent: "Black holes warp space-time and nothing can escape their gravity." }),
new Document({ pageContent: "The law of inertia is part of Newton's first law of motion." }),
];
// Step 2: Create a vector store
const vectorStore = await MemoryVectorStore.fromDocuments(docs, new OpenAIEmbeddings());
// Step 3: Set up the LLM
const llm = new ChatOpenAI({
modelName: "gpt-3.5-turbo",
temperature: 0,
openAIApiKey: process.env.OPENAI_API_KEY!,
});
// Step 4: Create the compression component using fromLLM
const baseCompressor = LLMChainExtractor.fromLLM(llm);
// Step 5: Create the ContextualCompressionRetriever
const retriever = new ContextualCompressionRetriever({
baseCompressor,
baseRetriever: vectorStore.asRetriever(),
});
// Step 6: Ask a question
const question = "What did Newton say about inertia?";
// Step 7: Retrieve compressed relevant info
const results = await retriever.invoke(question);
console.log("
🔍 Filtered / Compressed Results:");
results.forEach((doc, i) => {
console.log(`
#${i + 1}: ${doc.pageContent}`);
});
}
run().catch(console.error);
Ensemble Retriever
Combines the results from multiple retrievers into a unified set, increasing robustness and recall.
╔════════════════════════════════════════════════════════════════════════════╗
║ 🌟 EDUCATIONAL EXAMPLE 🌟 ║
║ ║
║ 📌 This is a minimal and short working example for educational purposes. ║
║ ⚠️ Not optimized for production! ║
║ ║
║ 📦 Versions Used: ║
║ - "@langchain/core": "^0.3.38" ║
║ - "@langchain/openai": "^0.4.2" ║
║ ║
║ 🔄 Note: LangChain is transitioning from a monolithic structure to a ║
║ modular package structure. Ensure compatibility with future updates. ║
╚════════════════════════════════════════════════════════════════════════════╝
import { ChatOpenAI } from "@langchain/openai";
import { OpenAIEmbeddings } from "@langchain/openai";
import { MemoryVectorStore } from "langchain/vectorstores/memory";
import { EnsembleRetriever } from "langchain/retrievers/ensemble";
import { MultiQueryRetriever } from "langchain/retrievers/multi_query";
import { Document } from "@langchain/core/documents";
import * as dotenv from "dotenv";
dotenv.config();
async function run() {
// Step 1: Sample documents
const docs = [
new Document({ pageContent: "Isaac Newton discovered gravity and described the laws of motion." }),
new Document({ pageContent: "Albert Einstein developed the theory of relativity." }),
new Document({ pageContent: "Quantum mechanics describes particles at the atomic scale." }),
new Document({ pageContent: "Black holes are regions in space with strong gravitational pull." }),
];
// Step 2: Create vector store
const vectorStore = await MemoryVectorStore.fromDocuments(docs, new OpenAIEmbeddings());
// Step 3: Create base retriever (normal vector search)
const baseRetriever = vectorStore.asRetriever();
// Step 4: Create a MultiQuery retriever (LLM reformulates the query)
const llm = new ChatOpenAI({
modelName: "gpt-3.5-turbo",
temperature: 0,
openAIApiKey: process.env.OPENAI_API_KEY!,
});
const multiQueryRetriever = await MultiQueryRetriever.fromLLM({
llm,
retriever: vectorStore.asRetriever(),
});
// Step 5: Create the EnsembleRetriever (combines multiple retrievers)
const ensembleRetriever = new EnsembleRetriever({
retrievers: [baseRetriever, multiQueryRetriever],
weights: [0.4, 0.6], // optional: set importance
});
// Step 6: Ask a question
const query = "What is known about gravity and black holes?";
// Step 7: Retrieve results (use invoke instead of deprecated getRelevantDocuments)
const results = await ensembleRetriever.invoke(query);
console.log("
📚 Ensemble Results:");
results.forEach((doc, i) => {
console.log(`
#${i + 1}: ${doc.pageContent}`);
});
}
run().catch(console.error);
Parent Document Retriever
ParentDocumentRetriever is a specialized retriever in LangChain that performs vector search on small, indexed child chunks, but returns the full parent document from which the chunk came. It’s ideal for use cases where maintaining full context and structure is important, such as long articles, transcripts, or reports.
╔════════════════════════════════════════════════════════════════════════════╗
║ 🌟 EDUCATIONAL EXAMPLE 🌟 ║
║ ║
║ 📌 This is a minimal and short working example for educational purposes. ║
║ ⚠️ Not optimized for production! ║
║ ║
║ 📦 Versions Used: ║
║ - "@langchain/core": "^0.3.38" ║
║ - "@langchain/openai": "^0.4.2" ║
║ ║
║ 🔄 Note: LangChain is transitioning from a monolithic structure to a ║
║ modular package structure. Ensure compatibility with future updates. ║
╚════════════════════════════════════════════════════════════════════════════╝
import { ChatOpenAI } from "@langchain/openai";
import { OpenAIEmbeddings } from "@langchain/openai";
import { RecursiveCharacterTextSplitter } from "@langchain/textsplitters";
import { ParentDocumentRetriever } from "langchain/retrievers/parent_document";
import { MemoryVectorStore } from "langchain/vectorstores/memory";
import { InMemoryStore } from "@langchain/core/stores";
import { Document } from "@langchain/core/documents";
import * as dotenv from "dotenv";
dotenv.config();
async function run() {
// Step 1: Sample documents
const docs = [
new Document({
pageContent: `
Isaac Newton made numerous contributions to science.
He formulated the laws of motion and universal gravitation.
His work laid the foundation for classical mechanics.
Newton also made significant advances in optics and invented calculus independently.
`,
}),
new Document({
pageContent: `
Albert Einstein developed the theory of relativity, fundamentally changing our understanding of space and time.
He introduced the famous equation E=mc^2 and received the Nobel Prize in Physics for his explanation of the photoelectric effect.
`,
}),
];
// Step 2: Create text splitters
const parentSplitter = new RecursiveCharacterTextSplitter({
chunkSize: 2000,
chunkOverlap: 200,
});
const childSplitter = new RecursiveCharacterTextSplitter({
chunkSize: 400,
chunkOverlap: 50,
});
// Step 3: Initialize vector store and docstore
const vectorstore = new MemoryVectorStore(new OpenAIEmbeddings());
const docstore = new InMemoryStore();
// Step 4: Create ParentDocumentRetriever
const retriever = new ParentDocumentRetriever({
vectorstore,
docstore,
parentSplitter,
childSplitter,
});
// Step 5: Add documents to the retriever
await retriever.addDocuments(docs);
// Step 6: Ask a question
const query = "What did Newton contribute to optics and motion?";
// Step 7: Retrieve relevant parent documents
const results = await retriever.invoke(query);
console.log("📄 Parent Document Results:");
results.forEach((doc, i) => {
console.log(`
#${i + 1}:
${doc.pageContent}`);
});
}
run().catch(console.error);
Self Query Retriever
Uses an LLM to translate a natural language question into a structured query, allowing more intelligent and dynamic retrieval from metadata-rich vector stores.
╔════════════════════════════════════════════════════════════════════════════╗
║ 🌟 EDUCATIONAL EXAMPLE 🌟 ║
║ ║
║ 📌 This is a minimal and short working example for educational purposes. ║
║ ⚠️ Not optimized for production! ║
║ ║
║ 📦 Versions Used: ║
║ - "@langchain/core": "^0.3.38" ║
║ - "@langchain/openai": "^0.4.2" ║
║ ║
║ 🔄 Note: LangChain is transitioning from a monolithic structure to a ║
║ modular package structure. Ensure compatibility with future updates. ║
╚════════════════════════════════════════════════════════════════════════════╝
import { ChatOpenAI } from "@langchain/openai";
import { OpenAIEmbeddings } from "@langchain/openai";
import { RecursiveCharacterTextSplitter } from "@langchain/textsplitters";
import { ParentDocumentRetriever } from "langchain/retrievers/parent_document";
import { MemoryVectorStore } from "langchain/vectorstores/memory";
import { InMemoryStore } from "@langchain/core/stores";
import { Document } from "@langchain/core/documents";
import * as dotenv from "dotenv";
dotenv.config();
async function run() {
// Step 1: Sample documents
const docs = [
new Document({
pageContent: `
Isaac Newton made numerous contributions to science.
He formulated the laws of motion and universal gravitation.
His work laid the foundation for classical mechanics.
Newton also made significant advances in optics and invented calculus independently.
`,
}),
new Document({
pageContent: `
Albert Einstein developed the theory of relativity, fundamentally changing our understanding of space and time.
He introduced the famous equation E=mc^2 and received the Nobel Prize in Physics for his explanation of the photoelectric effect.
`,
}),
];
// Step 2: Create text splitters
const parentSplitter = new RecursiveCharacterTextSplitter({
chunkSize: 2000,
chunkOverlap: 200,
});
const childSplitter = new RecursiveCharacterTextSplitter({
chunkSize: 400,
chunkOverlap: 50,
});
// Step 3: Initialize vector store and docstore
const vectorstore = new MemoryVectorStore(new OpenAIEmbeddings());
const docstore = new InMemoryStore();
// Step 4: Create ParentDocumentRetriever
const retriever = new ParentDocumentRetriever({
vectorstore,
docstore,
parentSplitter,
childSplitter,
});
// Step 5: Add documents to the retriever
await retriever.addDocuments(docs);
// Step 6: Ask a question
const query = "What did Newton contribute to optics and motion?";
// Step 7: Retrieve relevant parent documents
const results = await retriever.invoke(query);
console.log("📄 Parent Document Results:");
results.forEach((doc, i) => {
console.log(`
#${i + 1}:
${doc.pageContent}`);
});
}
run().catch(console.error);
Time Weighted Vector Store Retriever
Prioritizes documents based on recency or time of last access, which is useful in applications where time sensitivity matters (e.g., chatbots, live systems).
╔════════════════════════════════════════════════════════════════════════════╗
║ 🌟 EDUCATIONAL EXAMPLE 🌟 ║
║ ║
║ 📌 This is a minimal and short working example for educational purposes. ║
║ ⚠️ Not optimized for production! ║
║ ║
║ 📦 Versions Used: ║
║ - "@langchain/core": "^0.3.38" ║
║ - "@langchain/openai": "^0.4.2" ║
║ ║
║ 🔄 Note: LangChain is transitioning from a monolithic structure to a ║
║ modular package structure. Ensure compatibility with future updates. ║
╚════════════════════════════════════════════════════════════════════════════╝
import { OpenAIEmbeddings } from "@langchain/openai";
import { TimeWeightedVectorStoreRetriever } from "langchain/retrievers/time_weighted";
import { MemoryVectorStore } from "langchain/vectorstores/memory";
import { Document } from "@langchain/core/documents";
import * as dotenv from "dotenv";
dotenv.config();
async function run() {
// Step 1: Initialize the vector store
const vectorStore = new MemoryVectorStore(new OpenAIEmbeddings());
// Step 2: Create the TimeWeightedVectorStoreRetriever
const retriever = new TimeWeightedVectorStoreRetriever({
vectorStore,
memoryStream: [],
decayRate: 0.01, // Adjust decay rate as needed
k: 2, // Number of documents to retrieve
});
// Step 3: Add documents with appropriate metadata
const now = Date.now();
await retriever.addDocuments([
new Document({
pageContent: "The Earth revolves around the Sun.",
metadata: { last_accessed_at: now - 1000 * 60 * 60 * 24 * 7 }, // 7 days ago
}),
new Document({
pageContent: "The Moon affects tides on Earth.",
metadata: { last_accessed_at: now - 1000 * 60 * 10 }, // 10 minutes ago
}),
new Document({
pageContent: "Jupiter is the largest planet in the solar system.",
metadata: { last_accessed_at: now }, // Now
}),
]);
// Step 4: Query the retriever
const query = "Tell me something about planets.";
const results = await retriever.invoke(query);
// Step 5: Display the results
console.log("
🕒 Time-Weighted Results:");
results.forEach((doc, i) => {
console.log(`
#${i + 1}: ${doc.pageContent}`);
});
}
run().catch(console.error);
Retrieval methods are essential in Retrieval-Augmented Generation (RAG) systems, allowing language models to access and reason over external, up-to-date, or domain-specific knowledge in real time.
Other Retrieval Chains
- Vector Database Retrieval: Integration with vector stores like Qdrant, Pinecone, Weaviate, Milvus, FAISS, etc., for similarity search. Storing and retrieving embeddings for documents or other data.
- ElasticSearch Retrieval: Use ElasticSearch for retrieving documents based on keyword or full-text search.
- BM25 Retrieval: Classic keyword-based document retrieval using term-frequency inverse document frequency (TF-IDF) or BM25 algorithms.
- SQL Database Retrieval: Query relational databases (e.g., MySQL, PostgreSQL, SQLite) for structured data retrieval.
- Text File or Directory Retrieval: Retrieve documents or data stored in plain text files or a directory.
- Hybrid Search Retrieval: Combines keyword-based and vector-based retrieval for enhanced performance.
- Custom Retrieval: Build your custom retrieval logic by implementing the Retriever interface.
- Google Drive Retrieval: Access documents stored in Google Drive.
- Notion Retrieval: Retrieve data from Notion databases and pages.
- Knowledge Graph Retrieval: Query knowledge graphs like Neo4j.
- Azure Cognitive Search: Azure's full-text search service for enterprise-level data retrieval.
- Document Loaders with Metadata Filtering: Filter and retrieve documents based on metadata tags.
- LangChain Index Retrieval: Use LangChain’s built-in document indexing and retrieval systems.
- ChatGPT Retrieval Plugin: A plugin to connect ChatGPT for retrieving specific datasets.
Conclusion
In the evolving landscape of AI and machine learning, LangChain Retrieval Chains offer an innovative way to bridge the gap between large datasets and intelligent applications. By seamlessly integrating language models with various retrieval mechanisms,LangChain enables developers to unlock valuable insights from structured and unstructured data with unparalleled efficiency.
Through practical examples like document querying and API retrievals, this tutorial highlights how LangChain can transform how we interact with data. These tools simplify complex tasks such as summarizing documents, answering user-specific queries, and retrieving relevant information from diverse sources like vector databases, REST APIs, and more.
The flexibility and scalability of LangChain Retrieval Chains empower developers to create AI-driven applications tailored to unique use cases—whether in healthcare, education, customer support, or beyond. As a result, they pave the way for more interactive, accurate, and adaptive AI systems that revolutionize data interaction and management.
By embracing LangChain, you can harness the power of advanced retrieval chains to build intelligent systems that not only process data but also provide meaningful, actionable insights. The possibilities are endless, and LangChain is your gateway to exploring them.