LangChain - Intro
Imagine having a virtual assistant that not only understands your commands but also learns from your interactions, creating personalized responses. Or envision a customer support chatbot that can handle complex queries with ease, delivering accurate and context-aware answers. This is the power of LangChain.
The video is in Bulgarian
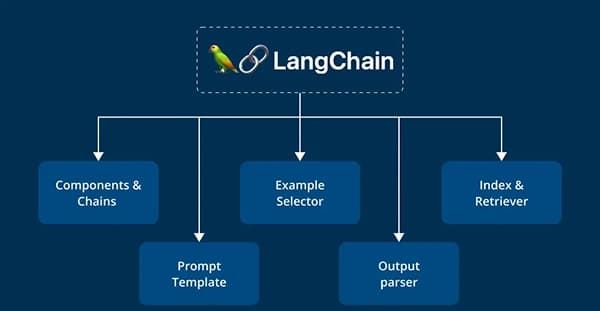
LangChain Introduction
With LangChain, you can automate the execution of actions, such as:
- 🔥 Automatically performing tasks, like reservations
- 🔥 Generating reports, articles, blog posts, or marketing materials
- 🔥 Automatically extracting key information from documents, such as contracts, scientific articles, or financial reports
- 🔥 Summarizing long documents or articles
- 🔥 Integrating with existing tools: connecting to databases like Qdrant, Pinecone, Redis, or libraries such as OpenAI, Hugging Face, or other models
LangSmith is a powerful developer platform by the creators of LangChain that helps you debug, test, and monitor LLM applications. It provides detailed traces of how your chains or agents behave, including which tools were called, what inputs were passed, and how long each step took—making it easier to understand, optimize, and improve your LLM workflows.
- 🔥 Debug and inspect your LangChain workflows in real-time with LangSmith
- 🔥 Track inputs, outputs, and tool usage inside your chains or agents
- 🔥 Identify bottlenecks, errors, and performance issues fast
- 🔥 Build production-grade LLM apps with better observability
LangGraph is a framework built on top of LangChain that lets you create stateful, multi-step AI applications using graphs instead of chains. It’s perfect for modeling complex workflows like agents that loop, branch, or revisit previous steps—something traditional chains can’t handle easily.
- 🔥 Model complex, non-linear workflows using graph-based logic
- 🔥 Build agents that can loop, branch, or revisit steps
- 🔥 Maintain state across interactions without custom code
- 🔥 Ideal for multi-agent collaboration, planning, or memory-based tasks
LangFlow is a visual programming interface for building LangChain applications. It lets you design and connect LLM components like tools, chains, memory, prompts, and agents using a drag-and-drop UI — no need to write full code to prototype or experiment!
- 🔥 Drag-and-drop UI to build LangChain apps visually
- 🔥 Connect components like LLMs, tools, memory, prompts, and chains
- 🔥 Instantly preview and test your logic without coding
- 🔥 Perfect for prototyping workflows and teaching LangChain concepts
Create the Project Directory
First, create the project directory and navigate into it:
mkdir langchain-tutorial
cd langchain-tutorial
Initialize the project with a package.json file:
npm init -y
This will generate a default package.json file. You will replace its content with the provided one in the next step.
Install TypeScript and the necessary types for Node.js:
npm install --save-dev typescript @types/node
Install ts-node and nodemon for Development. These tools help you run TypeScript files directly and automatically restart the server when files change:
npm install --save-dev ts-node nodemon
Install Express, a popular Node.js web framework, and its TypeScript definitions:
npm install express
npm install --save-dev @types/express
Install the langchain package and the OpenAI integration:
npm install langchain @langchain/openai
Install dotenv for environment variable management:
npm install dotenv
Create a tsconfig.json file to configure TypeScript:
npx tsc --init
Create the necessary directories and a basic index.ts file:
mkdir src
touch src/index.ts
Execution Method Invoke
This code sets up and runs a simple LangChain-based application that generates a joke using the OpenAI GPT-3.5-turbo model. It demonstrates how to:
- Load environment variables.
- Create and configure an OpenAI language model.
- Define a prompt template with a placeholder.
- Create a processing chain by piping the prompt into the model.
- Invoke the chain with a specific input and log the generated response.
╔════════════════════════════════════════════════════════════════════════════╗
║ 🌟 EDUCATIONAL EXAMPLE 🌟 ║
║ ║
║ 📌 This is a minimal and short working example for educational purposes. ║
║ ⚠️ Not optimized for production! ║
║ ║
║ 📦 Versions Used: ║
║ - "@langchain/core": "^0.3.38" ║
║ - "@langchain/openai": "^0.4.2" ║
║ ║
║ 🔄 Note: LangChain is transitioning from a monolithic structure to a ║
║ modular package structure. Ensure compatibility with future updates. ║
╚════════════════════════════════════════════════════════════════════════════╝
// src/index.ts
import { ChatOpenAI } from "@langchain/openai";
import * as dotenv from 'dotenv'
import { ChatPromptTemplate } from "@langchain/core/prompts"
dotenv.config()
async function main() {
// Create model
const model = new ChatOpenAI({
openAIApiKey: process.env.OPENAI_API_KEY!,
modelName: 'gpt-3.5-turbo',
temperature: 0.7
});
const prompt = ChatPromptTemplate.fromTemplate("You are a comedian. Tell a joke based on the following word {input}");
// Create chain
const chain = prompt.pipe(model)
// Call chain
const response = await chain.invoke({
input: "cat"
})
console.log(response)
}
main().catch(console.error);
Create model: An instance of ChatOpenAI is created with the following parameters:- openAIApiKey: This gets the OpenAI API key from environment variables.
- modelName: Specifies the model to use (in this case, gpt-3.5-turbo).
- temperature: Sets the creativity of the responses. A value of 0.7 means moderately creative responses.
- Create Chain:The prompt template is piped into the model using the pipe method. This creates a processing chain where the formatted prompt is directly fed into the language model.
- Invoke Chain:The chain is called (invoked) with the input "cat". This means the input word "cat" will replace the {input} placeholder in the prompt template. The formatted prompt is then processed by the model to generate a response.
- Run main Function:The main function is called and any errors are caught and logged to the console using console.error.
Streaming AI Responses in the Terminal with LangChain
╔════════════════════════════════════════════════════════════════════════════╗
║ 🌟 EDUCATIONAL EXAMPLE 🌟 ║
║ ║
║ 📌 This is a minimal and short working example for educational purposes. ║
║ ⚠️ Not optimized for production! ║
║ ║
║ 📦 Versions Used: ║
║ - "@langchain/core": "^0.3.38" ║
║ - "@langchain/openai": "^0.4.2" ║
║ ║
║ 🔄 Note: LangChain is transitioning from a monolithic structure to a ║
║ modular package structure. Ensure compatibility with future updates. ║
╚════════════════════════════════════════════════════════════════════════════╝
// src/index.ts
import { ChatOpenAI } from "@langchain/openai"; // used to create the model
import dotenv from "dotenv"; // used to load environment variables
dotenv.config(); // load environment variables from .env file
const model = new ChatOpenAI({ // create the model with key and model name, and temperature
openAIApiKey: process.env.OPENAI_API_KEY!,
modelName: "gpt-3.5-turbo",
temperature: 0.7,
})
async function run() {
const response = await model.stream("What are quarks in quantum physics?") // send the question to the model
process.stdout.write("🤖 AI: ") // Start response on the same line
for await (const chunk of response) { // for each chunk of the response
// checks if the content is an array, and joins the array elements with an empty string, or converts the content to a string
process.stdout.write(Array.isArray(chunk.content) ? chunk.content.join("") : String(chunk.content)
)
}
process.stdout.write("") // Move to a new line after completion
}
run().catch(console.error); // run the function and catch any errors
This script demonstrates how to interact with OpenAI’s GPT-3.5-turbo model using LangChain while streaming responses dynamically in the terminal. Unlike a standard API call that waits for the entire response before displaying it, streaming allows the response to appear word by word, simulating real-time typing.
How It Works
- Model Initialization The script initializes a ChatOpenAI model with the specified API key, model name, and temperature (which controls randomness in responses). The API key is loaded securely from environment variables using dotenv.
- Streaming the AI Response The model.stream() function sends a question to the AI model, requesting information about quarks in quantum physics. Instead of waiting for the full response, each chunk of data is processed as soon as it arrives.
- Efficient Terminal Output Handling process.stdout.write("🤖 AI: ") ensures that the response starts on the same line, rather than jumping to a new one.The for-await loop processes the response chunk by chunk, checking whether the content is an array or a string. The AI's words appear in real-time, creating a smooth user experience.
- Error Handling The script includes .catch(console.error) to catch and display errors if something goes wrong.
Summary
This code snippet is a simple demonstration of how to interact with OpenAI's language models using the LangChain framework. It loads an API key from an environment variable, sends a prompt to the OpenAI model, and then streams the response back, printing each chunk of the response (in this case, a poem about AI) to the console in real-time. This approach is particularly useful for handling long responses or when you want to process data as it arrives rather than waiting for the entire response.
Execution Method Batch
╔════════════════════════════════════════════════════════════════════════════╗
║ 🌟 EDUCATIONAL EXAMPLE 🌟 ║
║ ║
║ 📌 This is a minimal and short working example for educational purposes. ║
║ ⚠️ Not optimized for production! ║
║ ║
║ 📦 Versions Used: ║
║ - "@langchain/core": "^0.3.38" ║
║ - "@langchain/openai": "^0.4.2" ║
║ ║
║ 🔄 Note: LangChain is transitioning from a monolithic structure to a ║
║ modular package structure. Ensure compatibility with future updates. ║
╚════════════════════════════════════════════════════════════════════════════╝
// src/index.ts
import { ChatOpenAI } from "@langchain/openai"; // ✅ Import the ChatOpenAI class from LangChain's OpenAI wrapper
import dotenv from "dotenv"; // ✅ Load environment variables from .env
dotenv.config(); // ✅ Initialize dotenv to access OPENAI_API_KEY
// ✅ Initialize the OpenAI chat model
const model = new ChatOpenAI({
openAIApiKey: process.env.OPENAI_API_KEY!, // 🔐 API key from .env
modelName: "gpt-3.5-turbo", // 📦 Specify the model
temperature: 0.7, // 🎯 Controls randomness (0 = deterministic, 1 = more creative)
});
async function run() {
// 🧠 List of user prompts to process in parallel
const inputs: string[] = [
"Hello!",
"How are you?",
"What is LangChain?",
"Tell me a fun fact about AI.",
];
// 🔄 Send all prompts at once using batch() for efficient parallel processing
const responses = await model.batch(inputs);
// 📦 Loop through each response and print it nicely
responses.forEach((response, i) => {
// 🧵 Handle if response.content is an array or plain string
const content = Array.isArray(response.content)
? response.content.map((item) => (typeof item === "string" ? item : JSON.stringify(item))).join("")
: String(response.content);
// 📤 Output the prompt and corresponding model response
console.log(`
🧾 Prompt: ${inputs[i]}`);
console.log(`🤖 Response: ${content}`);
});
}
// 🏁 Run the function and catch any unexpected errors
run().catch(console.error);
Create model: An instance of ChatOpenAI is created with the following parameters:- async function run(): This defines an asynchronous function named run. The async keyword allows the function to use the await keyword, which is used to wait for asynchronous operations to complete.
- model.batch(["Hello", "How are you ?"]): The batch method sends multiple inputs (in this case, ["Hello", "How are you ?"]) to the language model at once and returns their responses. It's used for processing multiple prompts efficiently in one go.
- await: The await keyword is used to pause the execution of the run function until the batch method returns a response. This makes sure the code doesn't move on to the next line until the responses are received.
This code is a simple example of how to use the LangChain library with OpenAI's language models. It imports necessary modules, loads environment variables, creates an instance of the ChatOpenAI class with an API key, and sends a batch of prompts to the model, printing out the responses.
Execution Method Generate
╔════════════════════════════════════════════════════════════════════════════╗
║ 🌟 EDUCATIONAL EXAMPLE 🌟 ║
║ ║
║ 📌 This is a minimal and short working example for educational purposes. ║
║ ⚠️ Not optimized for production! ║
║ ║
║ 📦 Versions Used: ║
║ - "@langchain/core": "^0.3.38" ║
║ - "@langchain/openai": "^0.4.2" ║
║ ║
║ 🔄 Note: LangChain is transitioning from a monolithic structure to a ║
║ modular package structure. Ensure compatibility with future updates. ║
╚════════════════════════════════════════════════════════════════════════════╝
import { ChatOpenAI } from "@langchain/openai";
import { HumanMessage } from "@langchain/core/messages";
import { ChatGeneration } from "@langchain/core/outputs";
import dotenv from "dotenv";
dotenv.config();
const model = new ChatOpenAI({
openAIApiKey: process.env.OPENAI_API_KEY!,
modelName: "gpt-3.5-turbo",
temperature: 0.7,
maxTokens: 60,
});
async function run() {
const prompt = [new HumanMessage("Suggest a name for a drone coffee delivery startup.")];
// We pass the same prompt 3 times (instead of using n: 3).
const result = await model.generate([prompt, prompt, prompt]);
result.generations.forEach((genList, index) => {
const chatGen = genList[0] as ChatGeneration;
console.log(`☕ Suggestion ${index + 1}: ${chatGen.message.content}`);
});
}
run().catch(console.error);
What is .generate()?
The .generate() method in LangChain is used to request multiple completions or structured outputs from a model, typically for a batch of inputs.
Unlike .invoke(), which processes a single input and returns one response, .generate() can handle multiple prompts at once and returns a richer, structured result — including the generated outputs, usage metadata, and other diagnostic details.
This method is especially useful when you want to:
- Compare multiple model outputs,
- Explore different variations (e.g., with randomness/temperature),
- Analyze token usage or generation steps.
Each output is returned as a structured object — usually containing .text for standard LLMs or .message.content for chat-based models.
🛠️ Use .generate() when you need more than just the final answer — like control, visibility, and analysis over how the model responds.
Additional Methods
In LangChain, different methods allow users to interact with models in various ways. These methods control how models process input and return output—synchronously, in batches, or via streaming. Here are the most common methods:
- model.invoke – Calls the model synchronously with a single input.
- model.batch – Processes multiple inputs in a batch (parallel execution).
- model.stream – Streams output token by token, useful for real-time applications.
- model.generate – generates completions for multiple prompts at once and returns rich structured results, including output text and metadata like token usage and log information. Useful when more control or analysis is needed beyond invoke().
- model.streamLog – Streams intermediate steps and the final output. Useful for debugging and execution tracing.
- model.streamEvents – Streams real-time events, including intermediate results and the final output.
- model.transform – Transforms a stream of inputs into a stream of outputs. Useful for processing async generators.
LangChain Patterns & Concepts
In addition to the standard execution methods, LangChain provides additional patterns and utilities for building complex workflows:
- Runnable Chains – LangChain allows pipelining models and tools together, forming multi-step workflows.
- Retrieval-Augmented Generation (RAG) – Commonly used for knowledge-augmented responses, combining LLMs with databases like Pinecone or Qdrant.
- Memory & Context Handling – Using ConversationSummaryMemory and ConversationTokenBufferMemory, LLMs can remember past interactions.
Conclusions
LangChain offers a powerful framework for building AI-powered applications that are dynamic, context-aware, and personalized. From setting up your project to leveraging advanced chaining methods, LangChain simplifies the process of integrating AI into your projects. Whether you're using synchronous calls, batch processing, or real-time streaming, LangChain provides the tools needed to unlock the full potential of models like OpenAI's GPT.
By following this guide, you now have a solid understanding of how to get started with LangChain, including setting up your environment, understanding prompt structures, and working with advanced techniques like chaining and batch processing. These skills will help you build smarter, more efficient applications capable of handling complex queries and delivering tailored user experiences.
As you move forward, don't stop experimenting. Explore additional methods, integrate LangChain into different workflows, and push the boundaries of what AI-powered applications can achieve. LangChain's flexibility and ease of use make it an essential tool for developers who want to innovate and stay ahead in the rapidly evolving world of AI.